Entwicklung KI-basierter virtueller Sensoren zur Prozesssteuerung
Development of AI-based virtual sensors for process control
Objective
Sensors for special applications have often high investment costs and huge implementation efforts. For example the cost for Brix-Plato sensor to measure the sugar content of wort (or mash) can be above 10.000,00 Euro. A similar situation we have with measuring the water content in coffee beans. These kind of sensors have often limited accuracy and need to be calibrated before each operation, which limits their use in applications.
The objective of this project is to develop virtual sensors and integrate them into a cyber physical system (CPS), while using the data from the process and the process control (context data). From the context data we want to deduct the physical values we are really interested in, such as sugar content (wort) or water content (coffee beans).
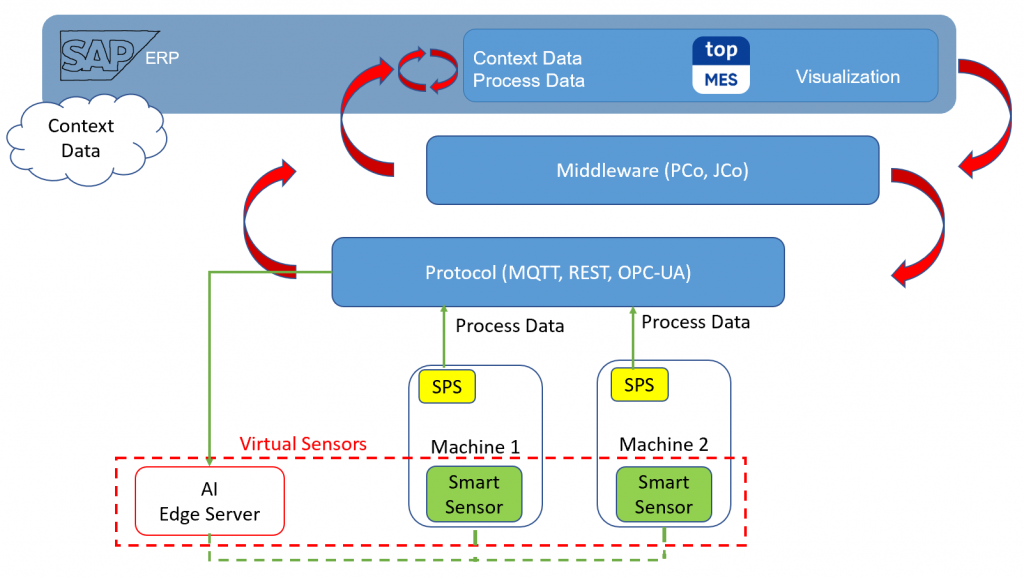
We have the following sub-goals:
- Development of virtual sensors integrated into a production process.
- Development of a demonstrator using a virtual sensor for a concrete application: Determining the sugar content during beer production
- Optimization of the process data during process control by bidirectional communication between virtual sensors and the enterprise planing system / manufacturing execution system (ERP/MES)
The virtual sensor will have inputs to measure process data and will have interfaces (such as MQTT) to other systems to request the process data and other context data. The virtual sensor computes the physical values we are interested in from the process data and context data with the help of AI. In Figure 1 you see the concept of the infrastructure of the complete CPS infrastructure.
We attach on the production plants (Machine 1, Machine 2 in Figure 1) a smart sensor which gathers the process data and pre-processes the data to reduce a amount of data. The smart sensor sends the pre-processed data to a AI-capable edge server, which is connected to the CPS. The edge sever is able to retrieve context data from the ERP/MES and computes with AI the interesting physical values, such as the sugar content of the wort (mash).
For training the AI model, the edge server gathers the process data from the production process and context data from the ERP/MES. While the ERP/MES sends control messages to the production system, the edge server listens to the messages and stores the control messages and the sensor data to a central influx database as a base for the AI training.
Team
In Figure 2 you find the company’s logos of the team members for the project KI-VISOPRO. The team leading institution is the University of Applied Science Albstadt-Sigmaringen (albsig). The KEIM institute is executing the project.
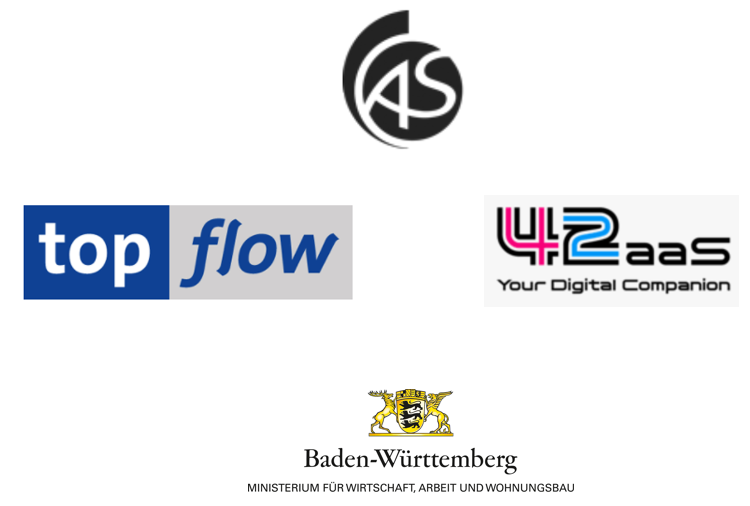
42aaS GmbH is a well experienced company in digital transformation and end to end solutions. It provides the perfect support in developing AI infrastructure for their customers, both from scratch or as digital retrofit at an existing set-up.
top flow GmbH is a SAP partner and focused on addon solutions for process digitalisation and automation. The manufacturing execution solution of top flow called top MES (see Figure 1), is a fully in SAP ERP and S/4HANA integrated standard software, which provides huge capabilities in interacting with SAP functions and context data.
The Ministerium für Wirtschaft, Arbeit und Wohnungsbau is sponsoring this project, see also Acknowledgement.
Acknowledgement
Special thanks to the University of Applied Science Albstadt-Sigmaringen for providing the infrastructure and the appliances.
This research is sponsored by the Ministerium für Wirtschaft, Arbeit und Wohnungsbau, Baden-Württemberg, see Figure 3. We are very thankful for providing us the financial support. The project KI-VISOPRO is part of the IW4.0 program. Further details can be retrieved from here.
